WHAT?
Two-day event on multi-modal neuroimaging! Lectures and hands-on on different neuroimaging types and their combination for 70 participants from engineers to doctors.
HOW?
Lectures and theory were given in the morning of each day. Participants were then able to apply the learned concepts during the afternoon hands-on sessions. All sessions were recorded and all resources have been created and made available, so that anyone can keep or start learning and using the tools! Check the lectures and tutorials!
WHY?
The main idea was to bring together participants from different backgrounds to learn about latest techniques on multi-modal neuroimaging integration, not only to possibly make new scientific advances but also to expand the horizon and perspectives of anyone involved!
Programme
The Sinergia Summer School 2021 took place online in GatherTown/Zoom over two days, from October Monday 11th to Tuesday 12th 2021.
Monday | Tuesday | ||
---|---|---|---|
8:30 | Introductory words by Patric Hagmann |
||
09:00 | Get to know each other |
||
9:30 | |||
10:00 | Morning break |
Morning break |
|
10:30 | |||
11:00 | |||
11:30 | |||
12:00 | Lunch break |
Lunch break |
|
13:00 | |||
14:00 | |||
15:00: | Afternoon break |
Afternoon break |
|
15:30 | |||
16:30 | Social event (TBD) |
Wrap up by Serge Vulliemoz |
Application
Application details
Applications have been now closed.
ECTS Credits
Participants who are (or will be) enrolled in PhD or Master programs can obtain ETCS credits for the participation in the Sinergia Summer School.
At the end of the school, 30 multiple choice questions have been sent as a course assessment task, for which the participant can respond online before October 30, 2021. Upon successful completion of the assessment, the participant will receive an "achievement" certificate over 1.5 ECTS for validation in your host program. If the participant "only" participate in the school without taking the final assessment, she/he will receive a "participation" certificate without credits.
Your opinion counts
At the end of the school, a link to an evaluation questionnaire has also been sent to know the (anonymous) opinion of the participants on the course for a second edition.
Lectures
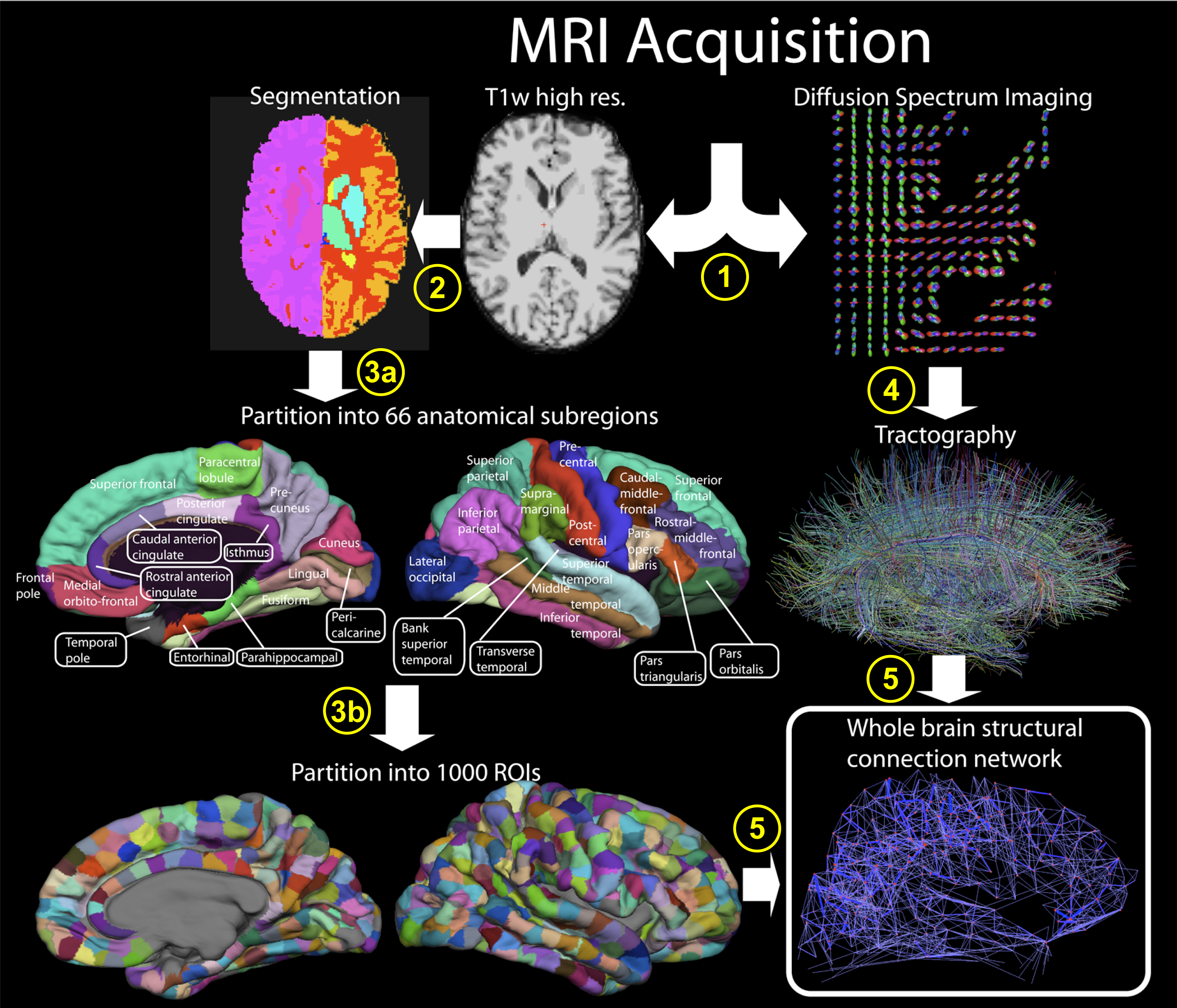
Lecture 1.1 - Building the Connectome
by Patric Hagmann
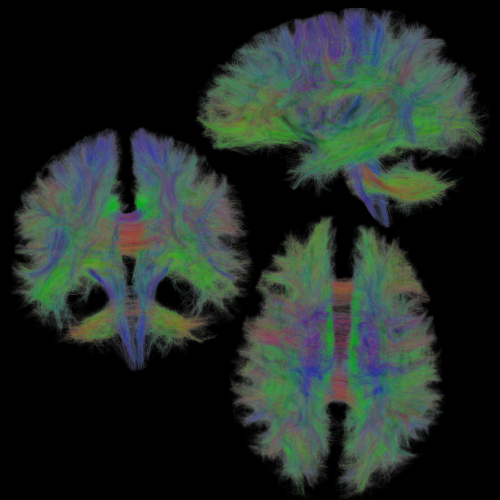
Lecture 1.2 - Diffusion and Tractography
by Marco Pizzolato
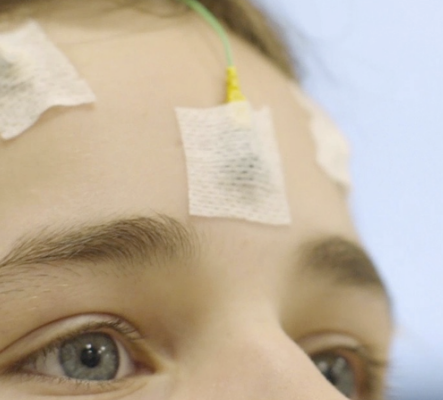
Lecture 1.3 - EEG Basics
by Serge Vulliemoz
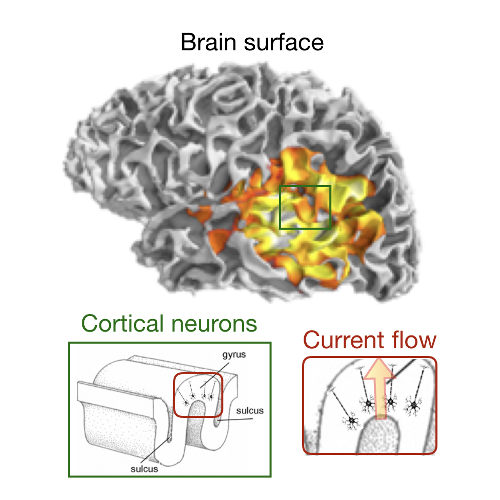
LECTURE 1.4 - Electrical Source Imaging
by Pieter van Mierlo
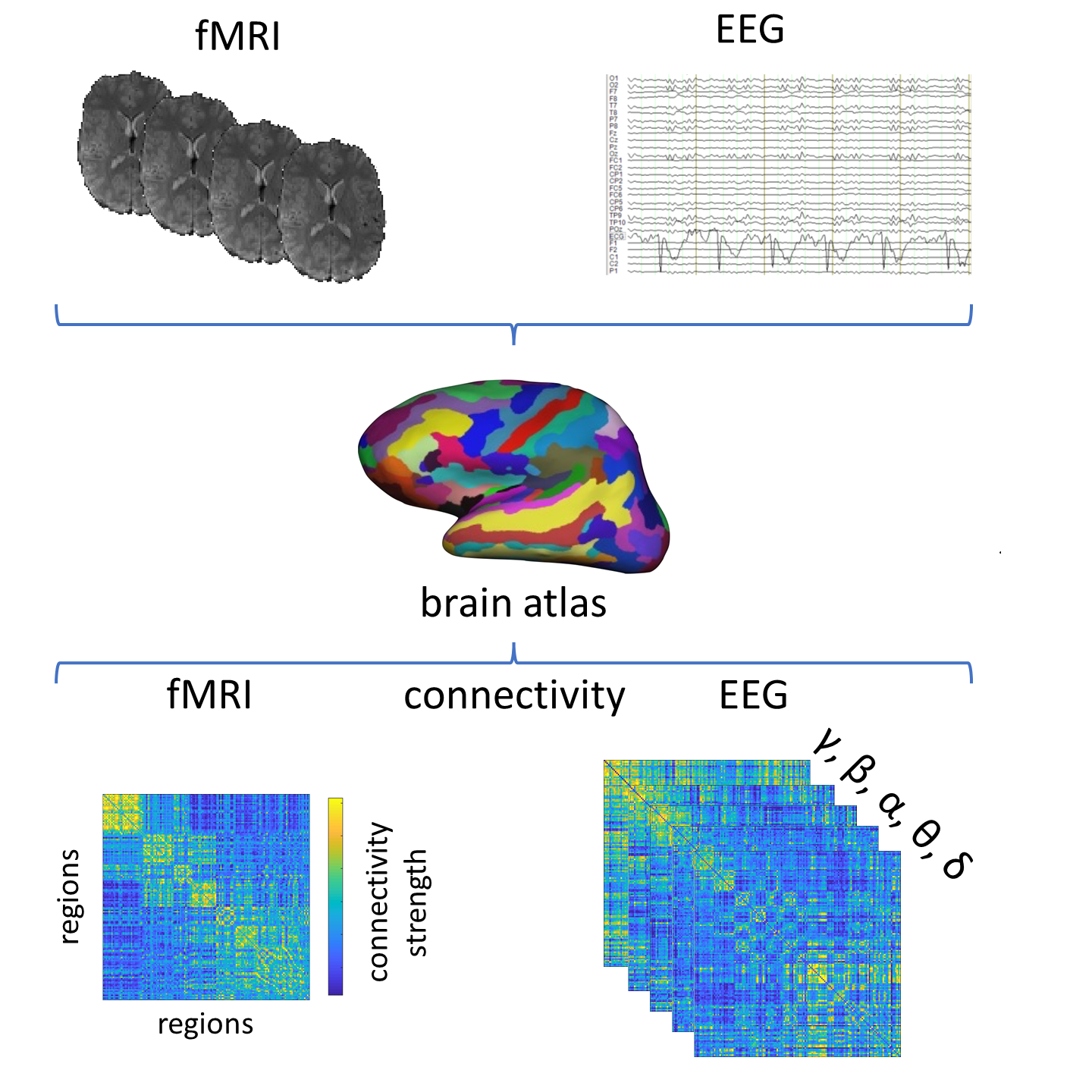
LECTURE 2.1 - Analysis of Brain Networks
by Jonathan Wirsich
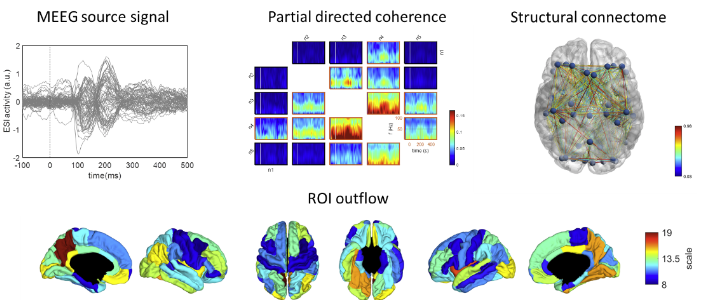
LECTURE 2.3 - Combining Structure and Function
by David Pascucci
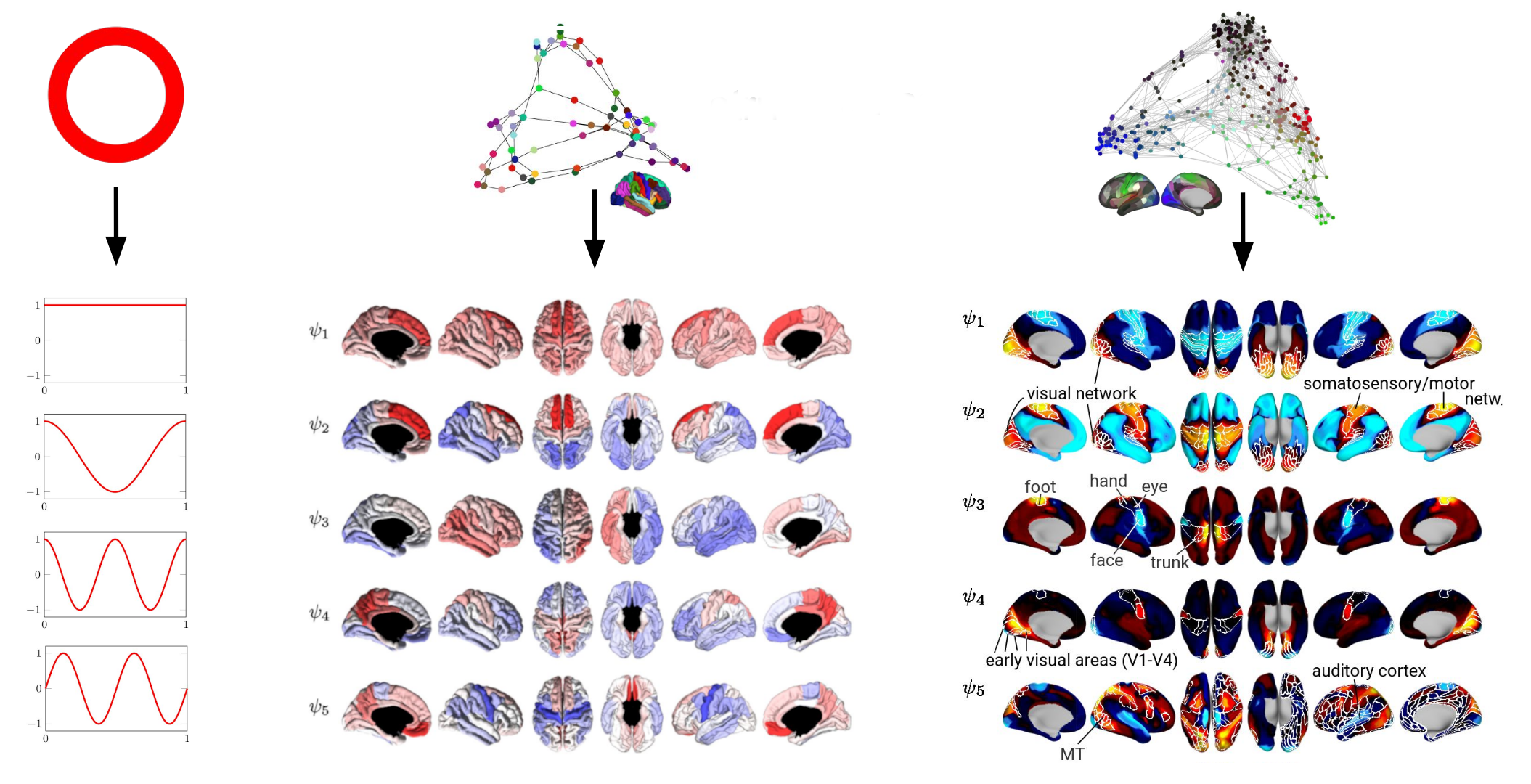
LECTURE 2.4 - Connectome Spectral Analysis
by Katharina Glomb
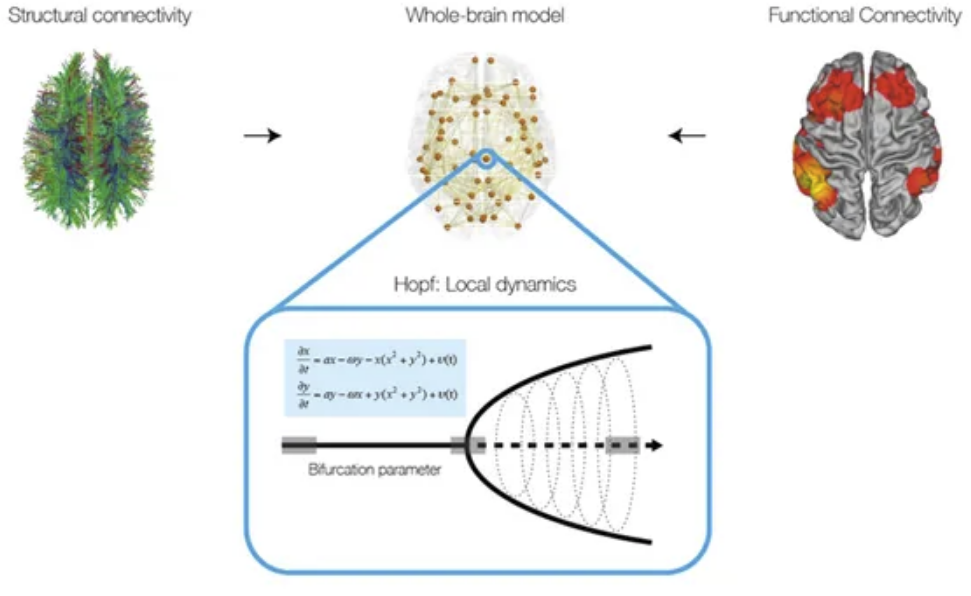
LECTURE 2.5 - Computational Modelling
by Gustavo Deco
Lecture 1.1 Building the Connectome by Patric Hagmann
In this talk I explain what is meant by «connectome» and how one can measure it with MRI.
- Link to slides.
Lecture 1.2 Diffusion and Tractography by Marco Pizzolato
The lecture covers the fundamentals of the diffusion MRI signal such as its interpretation, physical meaning, and directional dependence. These concepts are exploited to formulate the tractography problem, and various popular solutions to it are presented.
- Link to slides.
Lecture 1.3 EEG Basics by Serge Vulliemoz
In this lecture, we review the neurophysiological principles of EEG recording, together with their applications in clinical practice and neuroscience research. Normal and pathological activities on scalp EEG in wakefulness and sleep, as well as common artefacts are presented. Principles of intracranial EEG are discussed.
- Link to slides.
Lecture 1.4 Electrical Source Imaging by Pieter van Mierlo
The lecture introduces you to the open problem of how to represent the time- and frequency-content carried by hundreds of dipoles with diverging orientation in each brain region of interest with one unique representative time-series. This will help you to understand how much ad-hoc assumptions and constraints can influence the accuracy of the results in manipulating brain signals.
Reference:
Rubega, Maria, et al. "Estimating EEG source dipole orientation based on singular-value decomposition for connectivity analysis." Brain topography 32.4 (2019): 704-719.
- Link to slides.
Lecture 2.1 Analysis of Brain Networks by Jonathan Wirsich
The discovery of a stable, whole-brain functional connectivity organization that is largely independent of external events has drastically extended our view of human brain function. However, this discovery has been primarily based on functional magnetic resonance imaging (fMRI). The role of this whole-brain organization in fast oscillation-based connectivity as measured, for example, by electroencephalography (EEG) and magnetoencephalography (MEG) is only beginning to emerge. This lecture puts into context studies of intrinsic connectivity and its whole-brain organization in EEG, MEG, and intracranial electrophysiology with a particular focus on direct comparisons to connectome studies in fMRI. Irrespective of temporal scale over four orders of magnitude, intrinsic neurophysiological connectivity shows spatial similarity to the connectivity organization commonly observed in fMRI. A shared structural connectivity basis and cross-frequency coupling are possible mechanisms contributing to this similarity. Acknowledging that a stable whole-brain organization governs long-range coupling across all timescales of neural processing motivates researchers to take “baseline” intrinsic connectivity into account when investigating brain-behavior associations, and further encourages more widespread exploration of functional connectomics approaches beyond fMRI by using EEG and MEG modalities.
Reference:
Intrinsic connectome organization across temporal scales: New insights from cross-modal approaches, Sepideh Sadaghiani and Jonathan Wirsich, Network Neuroscience 2020 4:1, 1-29
- Link to slides.
Lecture 2.2 Functional Connectivity (FC) and Dynamical FC by Gijs Plomp
Perception, cognition and behavior critically depend on how multiple brain areas flexibly interact and form functional networks. In task situations, stimulus-evoked responses recorded with M/EEG reflect coordinated activity among multiple brain areas within 100 ms that show complex evolutions. This seminar introduces time- and frequency-resolved functional connectivity analyses of stimulus-evoked responses using multivariate autoregressive modeling. It presents the key concepts and challenges for deriving dynamic networks from ERP data at the brain’s native time scale, and highlights recent findings obtained with fast dynamic network modeling of large-scale sensory and cognitive processes.
- Link to slides.
Lecture 2.3 Combining Structure and Function by David Pascucci
In the last decade, the emerging field of network neuroscience has opened a new frontier of research into the structural and functional organization of human brain networks. Despite the inherent link between the two, structural and functional connectivity have been mostly investigated separately or compared against each other, revealing a rather complex relationship. On the one hand, indeed, structural properties like the topology, length and myelination of axonal pathways provide a static backbone for neuronal communication. On the other hand, functional interactions are highly dynamic and exploit multiple configurations of structural links at the sub-second time scale of sensory, motor and cognitive processes. In the present lecture, we discuss the concordant and discordant attributes of structural and functional brain networks and we introduce a new algorithm for combining the two in the context of dynamic connectivity of event-related M/EEG signals. We demonstrate how different structural properties can be incorporated as priors to inform time-varying directed connectivity analysis of M/EEG data in source space. This will help you to familiarize with advanced techniques for high-temporal resolution and multimodal connectivity analysis.
Reference:
Pascucci, D., Rubega, M., & Plomp, G. (2020). Modeling time-varying brain networks with a self-tuning optimized Kalman filter. Plos Biology.
- Link to slides.
Lecture 2.4 Connectome Spectral Analysis by Katharina Glomb
It is an intuitively understandable idea to view brain activity as a signal that spreads through a network of interconnected brain regions. Mathematically, such a network is also known as a graph, and apart from the brain, many things outside of neuroscience can be considered graphs: traffic networks, social networks, the irregular surfaces of 3-dimensional objects, and many more. Importantly, in this context, there is a distinction to be made between the signal - in the brain, this is neural activity - and the graph on top of which this signal plays out. This point of view makes it possible to apply tools from a well-established framework to neural data, i.e., graph signal processing (GSP). In this lecture, we take a look at how GSP can be used to decompose, interpret and statistically analyze neural signals on a graph.
- Link to slides.
Lecture 2.5 Computational Modelling by Gustavo Deco
- Link to slides.
Tutorials
Tutorials of the summerschool are hosted on their own dedicated webpages: https://sinergia-connectomics-summerschool-2021.github.io/scss21-training/.
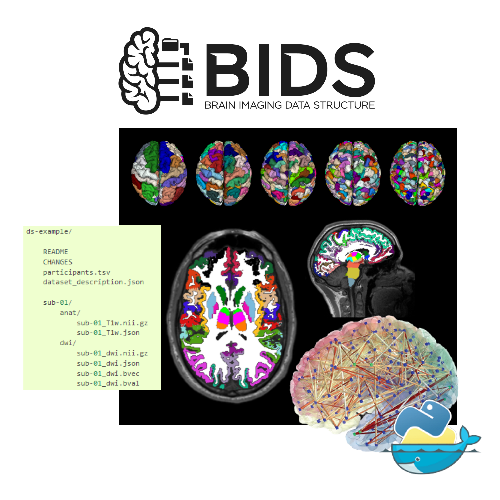
Tutorial 1 - Brain Imaging Data Structure
by Sebastien Tourbier
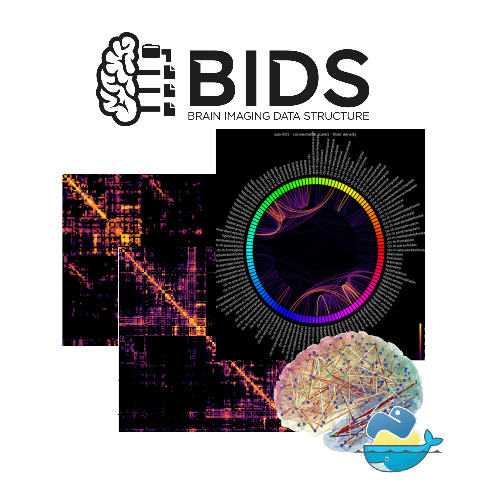
Tutorial 2 - Anatomical and Diffusion MRI pipelines
by Sebastien Tourbier
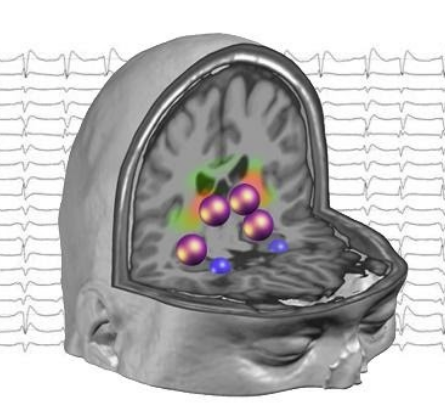
Tutorial 3 - Computing EEG time-series in brain regions
by Isotta Rigoni & Nicolas Roehri
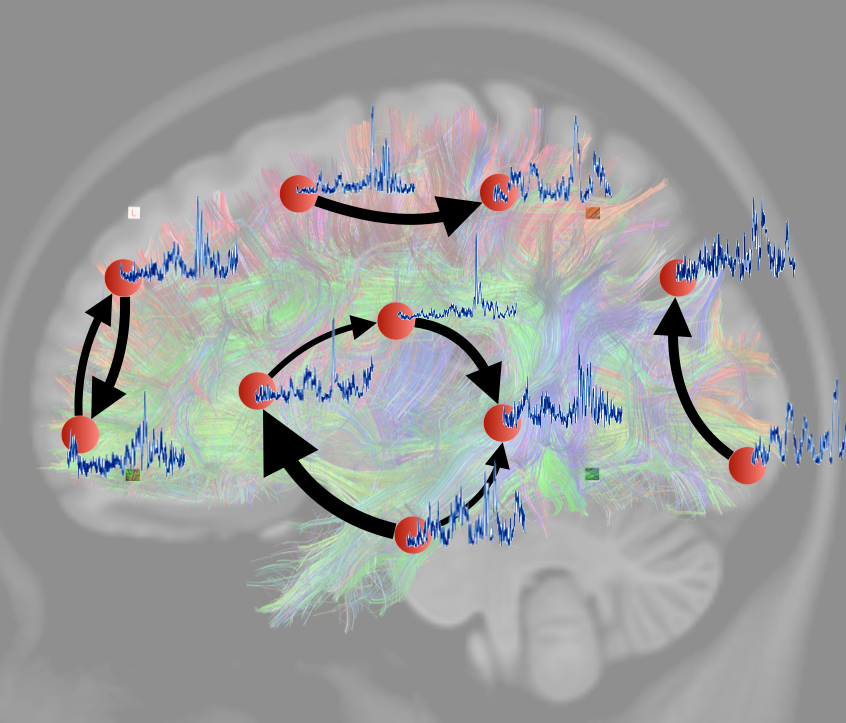
Tutorial 4 - Dynamic Functional Connectivity
by Maria Rubega & Jolan Heyse
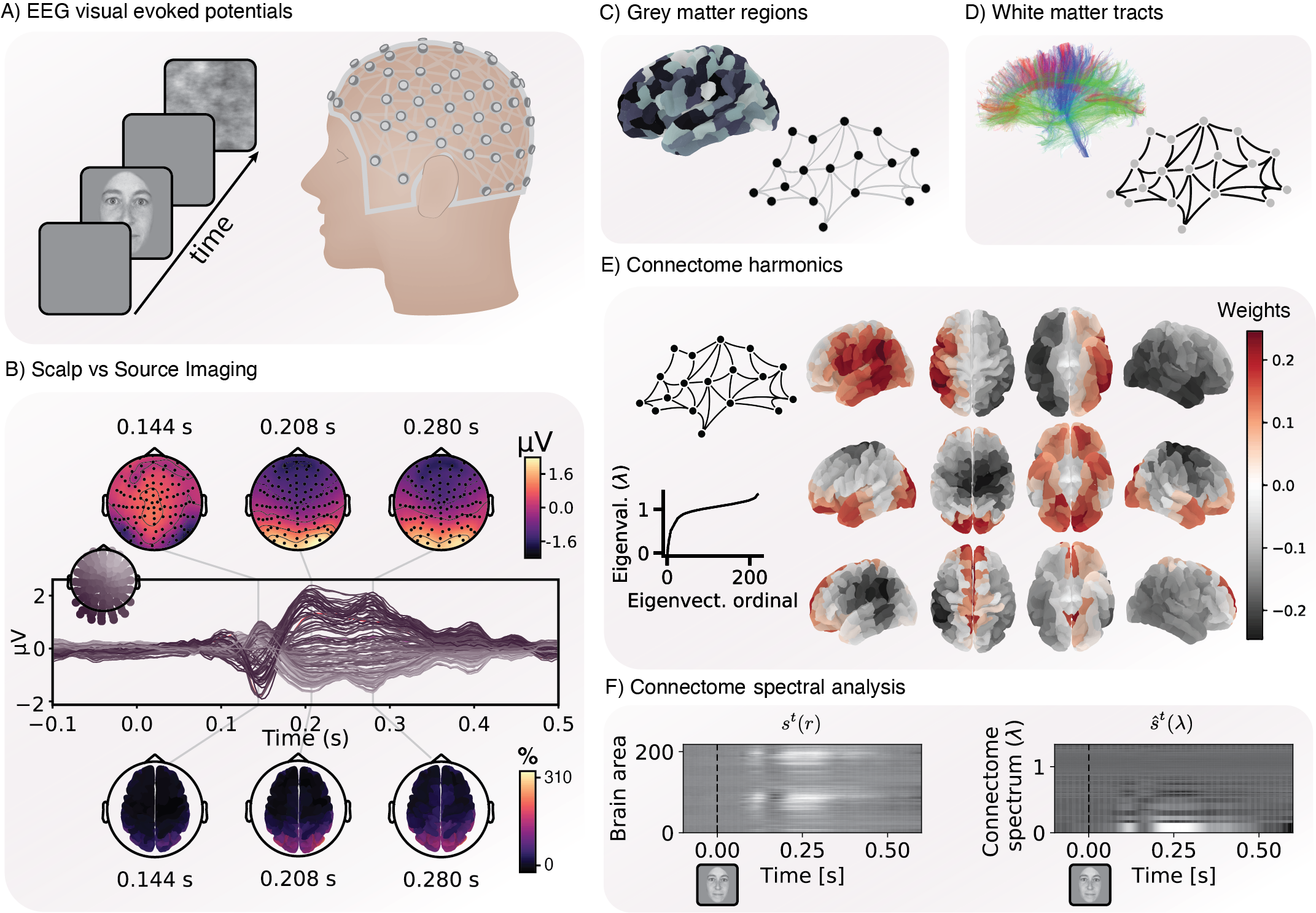
Tutorial 5 - Connectome Spectral Analysis
by Joan Rue-Queralt & Katharina Glomb
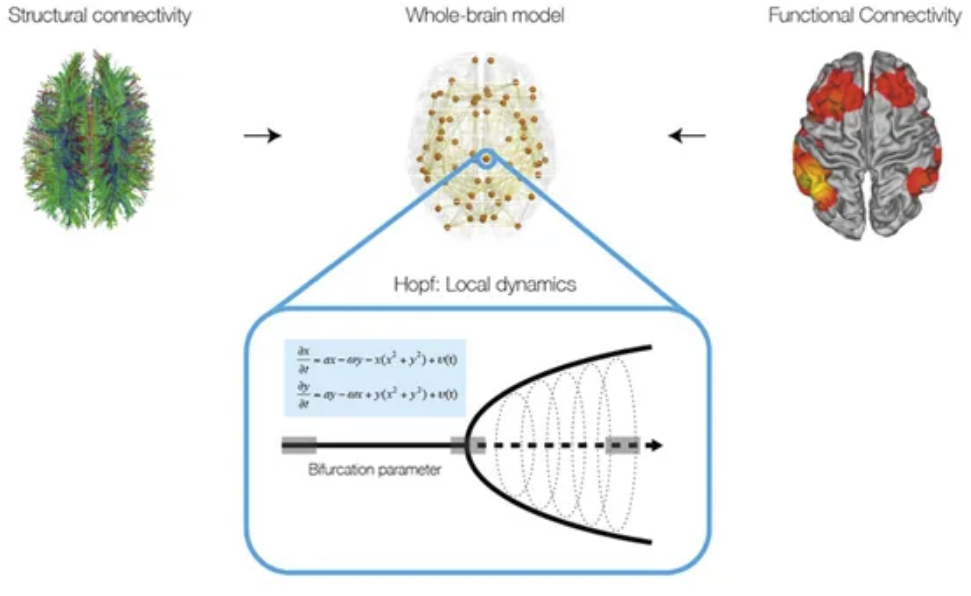
Tutorial 6 - Computational Modelling
by Ane Lopez-Gonzalez & Manel Vila-Vidal
Tutorial 1 Brain Imaging Data Structure by Sébastien Tourbier
The dataset that is used throughout most of the tutorials of the summer school follows the Brain Imaging Data Structure (BIDS) standard. This tutorial introduces you to BIDS and its eco-system.
- Link to tutorial webpage.
Tutorial 2 Computing structural connectivity matrices from diffusion MRI with a BIDS App by Sébastien Tourbier
The tutorial allows you to get more familiar with a BIDS App. We use the BIDS dataset introduced in Tutorial 1 and we guide you in all the steps involved in the computation of structural connectivity matrices derived from anatomical and diffusion MRI using a BIDS App: the Connectome Mapper 3. In particular, this includes: multi-scale brain parcellation based on the anatomical MRI, pre-processing of the diffusion MRI, T1w/parcellation/diffusion space co-registration, diffusion signal modeling, tractography and creation of structural connectivity matrices for each parcellation scale.
- Link to tutorial webpage.
Tutorial 3 EEG: from scalp to sources by Isotta Rigoni and Nicolas Roehri
The tutorial gives you hands-on starting from raw EEG data to representative time-series in each brain region of interest. This will allow you to have a general overview about different inverse methods for EEG source reconstruction.
Reference:
Rubega, Maria, et al. "Estimating EEG source dipole orientation based on singular-value decomposition for connectivity analysis." Brain topography 32.4 (2019): 704-719
- Link to tutorial webpage.
Tutorial 4 Dynamic Functional Connectivity by Maria Rubega and Jolan Heyse
The combination of structural and functional connectivity can shed new light upon the operational principles of brain networks. In this tutorial session we provide some hands-on experience in multimodal integration from the structural and functional networks. Starting from the structural connectome and source-reconstructed activity signals that were obtained in the previous tutorials, you will create a structurally constrained dynamic model of the brain networks. This is done by introducing the information from tractography (diffusion MRI) into a state-of-the-art measure for dynamic functional connectivity (Pascucci, D., Rubega, M., & Plomp, G. (2020). Modeling time-varying brain networks with a self-tuning optimized Kalman filter. Plos Bio). This tutorial requires some Matlab programming skills and a good understanding of the theoretic principles that were introduced during the lectures.
- Link to tutorial webpage.
Tutorial 5 Connectome Spectral Analysis by Joan Rue-Queralt and Katharina Glomb
In this tutorial we take one step further on the integration between EEG and dMRI by means of Connectome Spectral Analysis. You will apply tools from graph signal processing to discover statistical functional and statistical properties of the brain electrical signal that are revealed by representing the signal in terms of structural connectivity modes.
References:
- Glomb, Katharina, et al. "Connectome spectral analysis to track EEG task dynamics on a subsecond scale." (2020) NeuroImage.
- Queralt, Joan Rue, et al. "The connectome spectrum as a canonical basis for a sparse representation of fast brain activity." (2021) bioRxiv.
- Link to tutorial webpage.
Tutorial 6 Computational modelling by Manel Vila-Vidal and Ane Lopez-Gonzalez
Computational brain network models have emerged as a powerful tool to investigate the dynamics of the human brain. This tutorial introduces you to the basics of whole-brain computational modelling, with the aim of understanding its functionality and applicability. Specifically, we introduce a whole-brain network model based on a very general neural mass model known as the normal form of a Hopf bifurcation. In the first part of the tutorial we focus on understanding fundamental properties of the Hopf oscillator. In particular, we investigate the effect of the bifurcation parameter in the local node dynamics (describing either noisy or oscillatory behaviour) and the role of the global coupling parameter in the emerging patterns of global connectivity. In the second part of the tutorial, you will learn how to use this model to gain insight into global brain dynamics. In particular, we will construct a whole-brain model using structural and functional neuroimaging data and we will then use this model to reveal fundamental network principles of large-scale brain activity observable by noninvasive neuroimaging.
References:
- Deco, G., Kringelbach, M. L., Jirsa, V. K., & Ritter, P. (2017). The dynamics of resting fluctuations in the brain: metastability and its dynamical cortical core. Scientific reports
- Saenger, V. M., Kahan, J., Foltynie, T., Friston, K., Aziz, T. Z., Green, A. L., ... & Mancini, L. (2017). Uncovering the underlying mechanisms and whole-brain dynamics of deep brain stimulation for Parkinson’s disease. Scientific reports
- Link to tutorial webpage.
Team
Here is the team, members of the Sinergia consortium on brain communication pathways. The project consists in exploring brain communication pathways by combining diffusion based quantitative structural connectivity and EEG source imaging with application to physiological and epileptic networks. See project website for more details.
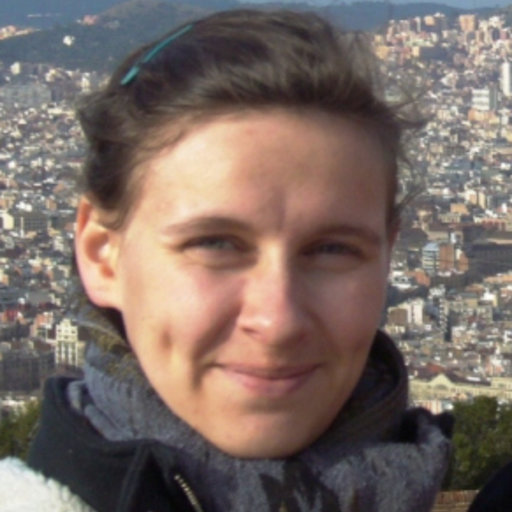
Katharina Glomb
Katharina's expertise: signal processing on connectome-based graph, combination of structural and functional connectivity
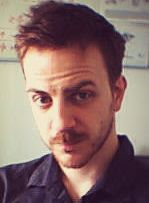
David Pascucci
David's expertise: dynamic causal functional connectivity, combination of structural and functional connectivity
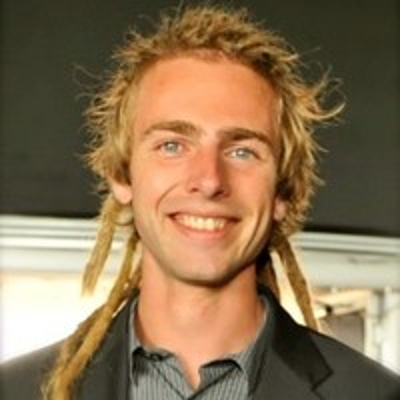
Sébastien Tourbier
Seb's expertise: medical image analysis, reproducible workflows, open-science, BIDS and BIDS App standards
Organizers
We are here to help. Don't hesitate to ask us any question.
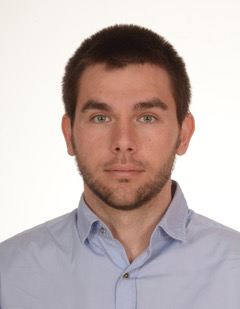
Joan Rué Queralt
Connectomics Lab, University Hospital of Lausanne (CHUV), Switzerland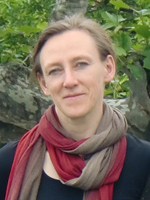
Ulrike Toepel
University of Lausanne, Switzerland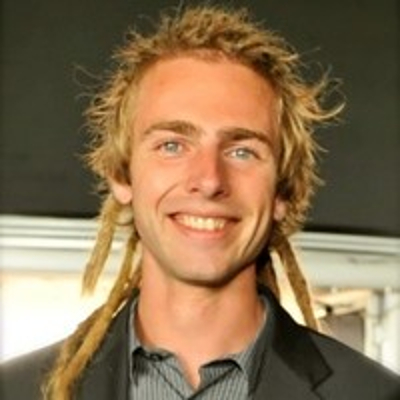